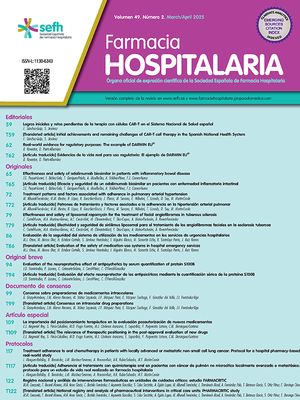
To perform a systematic review of studies assessing the impact of mHealth on treatment adherence in diabetes mellitus type II patients, describe target populations, and assess impact on adherence according to the approach and type of intervention implemented.
MethodA systematic review was carried out based on a literature search on scientific databases (Medline, Embase, Web of Science and Google Scholar) to identify clinical trials or quasi-experimental studies published between 2011 and 2021 assessing the impact of mHealth strategies on adherence to drug therapy in diabetes mellitus type II patients. A bias analysis was carried out of the studies identified based on risk-of-bias 2 and risk-of-bias in nonrandomized studies of interventions criteria.
ResultsOf the 120 studies retrieved, eight were included in the systematic review. The strategies identified included the use of medication reminders or educational notes sent via text messages; electronic dispensing systems; phone calls; automated response systems; and mobile applications. The impact of each strategy on adherence to drug therapy was variable, and positive results were obtained with the electronic dispensing system when used in combination with text messages, phone calls or mobile applications. Interventions were behavioral and/or educational. Inclusion criteria were homogeneous, with study patients having similar mean age, but with heterogeneous or unknown socioeconomic and educational level. Bias analysis raised some concerns in the totality of the studies included.
ConclusionsThe heterogeneity of results prevents firm conclusions from being drawn about the mHealth strategy that best improves adherence of diabetes mellitus type II patients to drug therapy. In most studies, patients older than 65 years are underrepresented, despite them accounting for 21% of diabetic patients in Spain. Interventions were behavioral and/or educational and are aligned with other publications on Telemedicine interventions. Further studies are needed to identify the most effective mHealth strategies in improving treatment adherence in diabetes mellitus type II patients.
Describir los estudios de mHealth publicados para mejorar la adherencia en pacientes con diabetes mellitus tipo II, su población objetivo y su impacto en función de la estrategia utilizada y el tipo de intervención.
MétodoSe realizó una revisión sistemática mediante búsqueda bibliográfica en bases de datos (Medline, Embase, Web of Science y Google Scholar) para identificar ensayos clínicos o cuasi experimentales publicados entre 2011 y 2021 que investigaran los efectos de estrategias de mHealth en la adherencia farmacológica de pacientes con diabetes mellitus tipo II. Se realizó un análisis de sesgo de los estudios encontrados según los criterios risk-of-bias 2 y risk-of-bias in nonrandomized studies of interventions.
ResultadosDe los 120 estudios identificados, ocho fueron incluidos en la revisión sistemática. Las estrategias encontradas fueron: sistemas de mensajes simples recordatorios o educacionales, sistemas de dispensador electrónico, llamadas telefónicas, sistemas automatizados de respuesta y aplicaciones para dispositivos móviles. El impacto sobre la adherencia fue variable, aunque se obtuvieron resultados positivos con sistemas de dispensador electrónico combinado con sistemas de mensajes simples, llamadas telefónicas y aplicaciones para dispositivos móviles. Los tipos de intervención encontrados fueron conductuales y/o educacionales. En cuanto a la población objetivo, los criterios de inclusión fueron homogéneos con una edad media similar, pero con niveles educativos y económicos heterogéneos o no registrados. El análisis de sesgo de los estudios planteó algunas preocupaciones para todos ellos.
ConclusionesLa heterogeneidad de resultados impide extraer conclusiones respecto a qué estrategia de mHealth mejora la adherencia farmacológica en pacientes con diabetes tipo II. La población mayor de 65 años está infrarrepresentada en la mayoría de los estudios, aunque suponen el 21% de los diabéticos en España. Los tipos de intervención encontrados son conductuales y/o educacionales, y están en línea con otras publicaciones sobre intervenciones de Telemedicina. Son necesarios más estudios para dilucidar qué estrategias de mHealth tienen un mayor impacto en la adherencia farmacológica en pacientes con diabetes mellitus tipo II.
Diabetes Mellitus type II (DM II) is the most common chronic metabolic disease in adults, affecting 463 million people in the world in 20191. By 2030, this figure is expected to progressively increase to 578 millions. DM II accounts for 90% of new diagnoses of diabetes, with a prevalence of 10% in the adult population in Spain1–3. In 2017, the prevalence of diabetes in Spain was higher in men and in patients older than 65 years, and increases dramatically from 75 years of age4.
The primary cause of morbidity and mortality in DM II patients is cardiovascular disease, and metabolic control is essential to reduce disease progression. A good metabolic control prevents progression of neuropathy, diabetic retinopathy and nephropathy5. It is estimated that 45% of patients with DM II do not attain their therapeutic target of glycemic control (HbA1c < 7%). Poor treatment adherence has been identified as one of the main contributing factors6.
The World Health Organization (WHO) defines adherence as “(…) the extent to which a person's behavior — taking medication, following a diet, and/or executing lifestyle changes — corresponds with the agreed recommendations from a healthcare provider.” Adherence to drug therapy is generally defined as “taking more than 80% of their prescribed medication”7. The rate of non-adherence to medication in adult and pediatric patients with DM II is estimated to reach 61%. This phenomenon is associated with factors related to the patient, practitioner, treatment, and healthcare system. The factors consistently associated with poor treatment adherence include lack of understanding on the disease and/or treatment; lack of social support; or comorbidities such as depression or cognitive deterioration, added to drug-related adverse events or high cost of the medication. Factors contributing to improving treatment adherence include fluent physician-patient communication and appropriate patient education8–12. Healthcare literacy achieved through health education is defined by the WHO as “the cognitive and social skills which determine the motivation and ability of individuals to gain access to, understand, and use information in ways that promote and maintain good health”13.
Poor adherence in DM II patients results in poor metabolic control, increased morbidity and mortality, higher frequency of ED visits and hospital admissions, and increased costs6.
The WHO defines Telemedicine as “The delivery of health care services, where distance is a critical factor, by all health care professionals using information and communication technologies for the exchange of valid information for diagnosis, treatment and prevention of disease and injuries, research and evaluation, and for the continuing education of health care providers, all in the interests of advancing the health of individuals and their communities”14. In patients with DM II, Telemedicine has been proven to favor patient autonomy and engagement, improve the monitoring of patients living in remote locations, contribute to enhancing the effectiveness of treatments, allow closer monitoring, and improve glycemic control and overall quality of life15–17. A range of interventions have been implemented to improve adherence, with differences in terms of the healthcare provider that designs or leads the intervention (nurse, pharmacist, robotic autonomous system …); the communication channel used to report information (in person, via mail, phone, or the Internet, among others); and the approach adopted (educational, technical, behavioral, mixed/hybrid)18–22.
According to the WHO, mHealth is “medical and public health practice supported by mobile devices, such as mobile phones, patient monitoring devices, personal digital assistants (PDAs), and other wireless devices”. In relation to the impact of mHealth on adherence to drug therapy, systematic reviews include studies involving patients with different diseases, with heterogeneous results23. Some studies assess the impact of different mHealth strategies (SMS, apps, phone calls …) on health outcomes in patients with DM II. However, these studies do not provide a description of the tools used and their impact on adherence to drug therapy24–28.
The incidence of DM II is increasing in the population groups that most frequently use information and communication technologies (ICTs), i.e. subjects aged 16 to 24 years29,30. ICTs are defined as “a set technologies that allow the elaboration, storage, recording and presentation of information in the form of voice, images and data contained in acoustic, optical or electromagnetic signals”31. In Spain, according to data from the National Statistics Institute, the availability of ICTs and use of the Internet in Spanish households have progressively increased since 2010. There is a mobile phone in virtually all households of the country (99.5%). The use of the Internet in the population with ages between 16 and 24 years is universal (99.8%) and decreases as children are younger. Around 89.5% of the population older than 55 years use the Internet, a percentage that decreases in the age group of 65-74 years, where use drops to 69.7%. Nevertheless, the use of the Internet has increased in all age groups, especially in the 65-74 year-old population, with a 6.1-point increase in 2021 with respect to 201930. Therefore, the implementation of mHealth strategies may have a significant impact on adherence to drug therapy in DM II patients.
In this context, a systematic review was of studies assessing the impact of mHealth on treatment adherence in DM II patients, describe target populations, and assess impact on adherence according to the approach and type of intervention implemented.
MethodsThis systematic review is based on PRISMA methodology32. A structured research question (PICO format) was used to identify the most appropriate search terms. Patients: diabetes type II. Intervention: any mHealth tool. Comparison: Any non-mHealth tools (Telemedicine was allowed). Results: impact on adherence to drug therapy.
Search strategyA search was conducted on PubMed, Embase, Web of Science and Google Scholar using the systematic review filter to identify articles that were published between March 1, 2011 and March 31, 2021. A prospective exploratory study demonstrated that these databases provide adequate coverage of references for literature searches in systematic reviews33.
The following MeSH search terms were used: 'telemedicine’ (and some Entry terms such as 'mobile health’, 'mhealth’, 'telehealth’); 'medication adherence’ (and the term 'drug adherence’); 'diabetes mellitus’; 'diabetes mellitus, type 2’. Emtree terms included: 'telemedicine’, 'diabetes mellitus’, 'non insulin dependent diabetes mellitus’ y 'medication compliance’. Other candidate terms not included in another more general Emtree term were also used, such as 'mHealth’, to extend search and increase sensitivity.
A variety of filters were used in literature search. In the case of Embase, results were restricted to controlle clinical trial and randomized clinical trial the Medline search was restricted to controlle clinical trial and randomized clinical trial; with regard to Web of Science, search was limited to “clinical trial”. In the case of Google Scholars, articles could not be filtered by type, although descriptors were required to appears in the title of the article.
Annex 1 details search synthesis in each database. Searches were carried out in the order specified using the terms described in the table.
Inclusion and exclusion criteriaWe included original peer-reviewed research papers which main intervention was based on the use of a mHealth tool, with the primary objective of assessing their impact on drug adherence in adult patients (> 18 years) with DM II receiving treatment with antidiabetic agents or other drugs to reduce cardiovascular risk. We searched experimental analytical studies (clinical trials) regardless of the blinding method used [simple, double, triple or none (open trial)] or quasi-experimental studies published either in English or Spanish.
We excluded observational, usability and acceptability studies testing mHealth apps, and experimental analytical studies that did not involve a mHealth-based intervention. In addition, studies in a sample of patients with conditions other than DM II when results for DM II patients were not reported separately were also excluded. Other studies excluded were systematic reviews, meta-analyses, editorials, comments, posters, abstracts and research protocol proposals.
Document classification and filteringMendeley Reference Manager® was used to classify references by source database and remove duplicates. Then, titles and abstracts were reviewed by the principal investigator for potential inclusion. The studies that complied with minimal inclusion criteria underwent full-text reading separately by two reviewers.
Data extractionThe following variables were identified in the studies included: type of study, sample size, mean age, sex distribution, economic and educational level of the subjects, mHealth tool used, description of the strategy and comparator, follow-up time and/or duration of the study, method used to measure adherence and the impact obtained (effect size) and type of intervention34.
The risk of bias (internal validity) in randomized clinical trials was assessed using the risk-of-bias (RoB-2) tool modified according to Cochrane collaboration35,36. Risk-of-bias in nonrandomized studies of interventions (ROBINS-I) scale was used for non-randomized trials, as it has been demonstrated to complement GRADE methodology when assessing the level of evidence in this type of studies37,38. This tool was used in other systematic reviews including one-arm trials39,40.
ResultsSelection of articlesFollowing the search strategy described above, 279 papers were retrieved, which were reduced to 120 papers after duplicates were removed. A total of 87 studies were excluded during abstract and title review for several reasons: 28 studies were excluded as they were not focused on adherence to non-insulin antidiabetic drugs (NIAD); 23 corresponded to research protocols without results published; 11 were qualitative evaluations of mHealth tools in development; 10 were not experimental or quasi-experimental studies; 4 did not test a mHealth-based intervention; and a study was excluded because it was not available in English or Spanish.
The remaining 33 did not undergo full-reading. Of them, 25 were ruled out. Thus, 8 papers met inclusion criteria and were selected for systematic review. The reasons for exclusion of the 25 papers from full-reading were: 14 were excluded because assessing adherence was not the primary objective; 8 because > 50% were not DM II patients; 2 were excluded as the use of a mHealth tool was not the main intervention, and one because it was a post-hoc analysis of another trial that had already been included in our review. The flowchart for the selection of reviewed articles based on the PRISM scheme is shown in figure 1.
mHealth strategies and impact on drug adherenceTwo studies used electronic dispensing systems combined with SMS medication reminders when the package was not opened within a specific time period41,42. This enabled real-time monitoring of adherence, unlike in other studies. Sugita et al. combined daily SMS reminders with SMS to improve health literacy in the intervention group, whereas controls only received SMS reminders43. Gatwood et al. tested a strategy based on tailored text messages to improve health literacy44. Text messages were sent to remind the patient about taking the first daily dose of NIAD. Mayberry et al. used SMS reminders that required response (YES/NO), combined with SMS aimed at reducing barriers to adherence45. In addition, the authors implemented a strategy based on weekly phone calls with an automated response system (ARS) that provided feedback to patients on their adherence in the last week, as well as indications to solve drug-related problems. Yasmin et al. used ARS-based medication reminders combined with lifestyle recommendations, and activated a 24/7 call center where patients could access a physician to get health-related information and suggestions46. The strategy implemented by Odegard et al. involved two phone calls from the community pharmacist separated by a period ranging from one week to one month. The authors used the guided script based on the “4 A's” (ask, advise, assist, and arrange) behavioral model to assess DM II management and improve drug adherence47. Finally, Huang et al. implemented a mobile app that generated tailored medication schemes and daily reminders. Barriers to medication adherence and self-appraisal of diabetes were assessed using the Adherence Starts with Knowledge-12 (ASK-12) questionnaire48.
The impact of these mHealth-based strategies on adherence to drug therapy was variable. Of the eight studies included in the review, the intervention improved drug adherence significantly in four studies41,42,47,48. In contrast, the intervention did not have any statistically significant effect on adherence in four studies43–46.
Raiff et al. measured adherence based on the percentage of doses taken within the therapeutic window established and number of days the patient took his/her medication. Adherence was assessed by Vervloet et al. based on the medication possession ratio (MPR), defined as the percentage of days covered with the medication dispensed in the refilling interval according to the percentage of prescriptions filled at the pharmacy. The two studies revealed an improvement in adherence, although Raiff et al. do not provide a statistical data analysis42. Vervloet et al. report a statistically significant difference between the incentive group with SMS and the control group, in terms of MPR at 12 months (MPR-12) [mean: 15.0% (6.7-23.4); p < 0.001] and MPR at 24 months (MPR-24) [mean: 12.0% (3.1-21.0); p < 0.01]. Intra-group adherence also improved in incentive plus SMS reminders [+16.3% (9.2-23.4); p < 0.001] and incentive strategy without SMS [+10.1% (2.2-18.1); P < 0.05] with respect to baseline, which was not observed in controls41.
Odegard et al. assessed adherence based on the days of late refill after the intervention and MPR at 6 and 12 months after the intervention, as compared to baseline values. The mean number of days for first refill of medication in the intervention group was lower than in controls (6.8 vs 8.5), although difference was not statistically significant (p = 0.37). Conversely, a statistically significant increase was observed in intra-group adherence in the intervention group, in terms of MPR-12 [0.85 vs 0.90 (p < 0.01)] (not observed in the control group), and a difference with respect to the control group, also significant in MPR-12 (p = 0.01)47. Adherence also increased with the mobile app-based strategy implemented by Huang et al., as a statistically significant difference of 4.7 points was observed on ASK-12 score [1.2-8.2 (p = 0.01)]48.
In turn, Gatwood et al. did not obtain any significant effect in drug adherence as measured based on days of medication available per refill at the pharmacy (p > 0.05)44. Mayberry et al. assessed adherence according to variations in score on the Summary of Diabetes Self-Care Activities (SDSCA) and responses to the daily SMS reminder. Although a within-subject reduction was found in some barriers to adherence, it did not result in significant variations in SDSC score or number of answers to SMS reminders45. Sugita et al. do not document statistically significant differences in drug adherence between the intervention and the control group, as measured on Morisky Medication Adherence (MMAS-8) scale at 6 months [11.8% vs 14.2% (p = 0.78)]43. This result was consistent with that of Yasmin et al., where self-reported adherence did not improve46.
Table 1 describes study designs, the strategies implemented, the adherence assessment tools used, and their impact on adherence.
mHealth strategies and impact on drug adherence
Study | Design (N) | Description of the strategy (duration) | Assessment of adherence | Impact on adherence |
---|---|---|---|---|
Odegard et al. 2012 | Randomized, placebo-controlled trial 1:1 (265) | Protocolized call based on the 4 A's model (ask, advise, assist, arrange) at weeks 0, 1 and 4. | Rate of refills (days of late refill) and MPR at 6 and 12 months pre-post intervention. | No significant in days of late refill (p = 0.37), % of first late refill (p = 0.70) or MPR-6 (p = 0,24). Significant in MPR-12 (p = 0.01). |
Raiff et al. 2012 | Pre-post quasi-experimental study 1 arm (3) | ED where an SMS reminder is sent if the patient does not open it in time. Economic reward for adherence from the third day. | ED data and need for SMS reminder pre-post intervention. | Increase in doses taken within therapeutic window and total pre-post adherence (50.0% vs 95.4%, 50.0% vs 91.3% and 0.0% vs 71.4%). |
Gatwood et al. 2016 | Randomized, placebo-controlled trial 1:1 (75) | Customized SMS according to the Health believe model and Self determination theory (90 days). | % change of days with medication available according to refills at the pharmacy. |
|
Mayberry et al. 2017 | Open, randomized, placebo-controlled trial 1 arm (80) | Tailored SMS following identification of barriers to adherence, and daily automatic calls (3 months). | Change in SDSCA questionnaire at months 0, 1, 2 and 3; answers to the SMS reminder. | No significant differences according to SDSCA (6.13 ± 1.22 vs 6.18 ± 1.35 days of weekly adherence), or in answers to SMS reminder (85.8 ± 20.5%). |
Vervloet et al. 2014 | Randomized, placebo-controlled trial 1:1:1 (161) | ED where an SMS reminder is sent if the patient does not open it within therapeutic window; group with ED without SMS reminders and control (24 months). | MPR at 12 and 24 months from initiation of study. | Significant difference with respect to control at 12 months [mean difference 15.0% (6.7-23.4); p < 0.001] and 24 months [12.0% (3.1-21.0); p < 0.01]. |
Sugita et al. 2017 | Open, randomized, placebo-controlled trial 1:1 (41) | SMS reminder of medication intake (in the two groups) + 2 weekly SMS for patient education (7 months). | Change on MMAS-8 scale at 6 months. | No significant. Rate of change on MMAS-8 of 11.8% and 14.2% in intervention and control groups, respectively (p = 0.78). |
Huang et al. 2019 | Randomized, placebo-controlled trial 1:1 (51) | Use of APP Medisafe with a pharmacological scheme (12 weeks). | Change on ASK-12 questionnaire at weeks 0 and 12 (ITT analysis) adjusted for years with diabetes and baseline ASK-12 score. | Significant: mean pre-post changes on ASK-12 of 4.7 points (1.2-8.2) lower in the intervention group vs control (p = 0.01). |
Yasmin et al. 2020 | Open, randomized, placebo-controlled trial 1:1 (320) | Tailored educational calls every 10 days + call center available 24/7 (12 months). | Difference in self-reported adherence defined as not missing a dose. | No significant pre-post differences (> 90% of adherence in all cases). |
ASK: Adherence starts with knowledge; ED: electronic dispenser; MMAS-8: Morinsky medication adherence scale-8; MPR: rate of refills at pharmacy; SDSCA: Summary of Diabetes Self-Care Activities; SMS: short message service.
From the point of view of the type of intervention, studies were homogeneous. Most studies involved a behavioral intervention aimed at changing or modifying patient behavior in relation to drug adherence and disease management41–45,47,48. In four studies, the strategy only consisted of a behavioral intervention, whereas another three studies combined the intervention with an educational intervention. Four studies included an educational intervention (in a mixed intervention in three studies and alone in another study)43,44,46,47. Therefore, of the eight studies reviewed, mHealth-based strategies were implemented in combination with other strategies in three studies43,44,47.
With regard to their impact on drug adherence, two studies involving mixed interventions reported non-significant differences, whereas the study by Odegard et al.47 revealed positive results. Adherence parameters improved in three of the four studies that only involved a behavioral intervention41,42,48. The study only including an educational intervention did not show any significant variations in self-reported adherence46.
Description of the target populationThe inclusion criteria applied in the different studies were quite homogeneous, as they included adult patients (> 18 years) having a mobile phone, without sensory disabilities, with proven lack of adherence based on refills at the pharmacy, validated questionnaire scores or a clinical parameter (HbA1c). Odegard et al. observed that the intervention was more effective in the population with lower baseline adherence (MPR < 0.8) based on MPR-6 and MPR-12, as compared to controls, although these parameters were not assessed in other studies47. Half of the studies excluded patients with cognitive impairment or mental illness, whereas the remainder did not provide any data on this variable43,45,47,48.
Mean age was similar, except for the study by Odegard et al, where mean age was 63 ± 13 years, slightly above mean age in the other studies47. Vervloet ef al. excluded patients older than 65 years, as they were less likely to use a mobile phone41.
Sex distribution was close to 50% in four studies. However, in the studies by Mayberry ef al. and Yasmin ef al., women accounted for 68% and 75% of the sample, respectively45,46. In contrast, in Sugita ef al. and Raiff ef al. studies, men accounted for 70% and 75% of the sample, although in the latter the sample consisted of 3 patients, which is not representative42,43.
Educational level was only assessed in three studies, being low (30-50%) in the studies by Vervloet ef al. and Huang ef a/.41,48 Yasmin ef al. report an educational level below or equal to secondary education in 90% of cases, although this study was conducted in Bangladesh, where literacy rates are generally low6. Income was assessed in four studies, being variable as different assessment methods were used. Huang ef a., in Singapur, report that 30-47% of subjects had an income < $4,00048. The subjects in the Gadwood ef al. study reported an annual income < $25,000 in 25-29.2% of cases, whereas 7-12% of cases in the Yasmin et al. study had a monthly income < €11644,46. Of note, 82% of cases in the Mayberry et al. study in USA had a low income (< $ 25,000/year), which was a barrier to adherence.
The follow-up period of the intervention was < 6 months in four studies, with a maximum of 24 months, in the study by Vervloet et al.Table 2 summarizes the types of interventions and profiles of target populations.
Type of intervention and target population
Study | Target population Inclusion criteria | Sample [mean age (years)/gender-based distribution] | Type of intervention |
---|---|---|---|
Odegard et al. 2012 | Patients > 18 years owning a phone without hearing/visual disability, without cognitive deterioration, with active prescription of NIAD and late refills at pharmacy > 6 days. |
| Educational Behavioral |
Raiff et al. 2012 | Patients 30-75 years with con DM II on treatment with NIAD for > 3 months. Level of HbA1C 7-10%, lack of adherence in the last 7 days, without known mental illness or pregnancy. |
| Behavioral |
Vervloet et al.. 2014 | 18-65 year-old patients with DM II > 1 year on NIAD, with a rate of refills < 80% the previous year, phone users. | Mean age = 55 55% men | Behavioral |
Gatwood et al. 2016 | Patients > 21 years with a level of HbA1C ≥ 8% with a record of not having taken ≥ 1 tabletsin the last 30 days. Without istory of ischemic events or heart insufficiency. | Age = 47 50% women | Behavioral Educational |
Mayberry et al. 2017 | Patients > 18 years with DM II on drug therapy, without sensory disability or cognitive impairment. | Mean age = 50.1 ± 10.5 68% women | Behavioral |
Sugita et al. 2017 | Patients > 18 years with DM II, HbA1C ≥ 6.5%, without depressive symptoms on QIDS-J scale, phone users. | Mean age = 56 70% men | Behavioral Educational |
Huang et al. 2019 | Patients with DM II > 21 years without cognitive or psychological disorders considered to be non-adherent according to ASK-12 questionnaire. |
| Behavioral |
Yasmin et al. 2020 | Patients with DM II on treatment, phone users. |
| Educational |
ASK-12: Adherence starts with knowledge-12; DM II: diabetes mellitus type 2; HbA1C: glycosylated hemoglobin; NIAD: non-insulin antidiabetics.
Risk assessment in randomized clinical trials raised some concerns, due to the fact that study subjects and health providers were not blind to the study arm the patient had been allocated to (risk of bias due to deviations from interventions). In non-randomized trials, risk-of-bias assessments revealed a high risk due to difficulties in completing evaluations and the lack of clarity in the explanation of participant selection procedures. Tables 3 and 4 show the results of risk-of-bias assessments in randomized and non-randomized studies, respectively.
Risk of bias in randomized trials according to RoB-2 criteria
Risk of bias in the randomization process | Risk of bias due to deviations from the interventions proposed | Risk of bias due to missing outcome | Risk of bias due to outcome measurement | Risk of bias due to reported outcome selection | Total risk of bias | |
---|---|---|---|---|---|---|
Gatwood et al. 2016 | Low | Low | Low | Low | Some concerns | Some concerns |
Huang et al. 2020 | Low | Some concerns | Low | Low | Low | Some concerns |
Odegard et al. 2012 | Some concerns | Low | Low | Low | Some concerns | Some concerns |
Sugita et al. 2017 | Low | Some concerns | Low | Low | Low | Some concerns |
Vervloet et al. 2014 | Low | Some concerns | Low | Low | Low | Some concerns |
Yasmin et al. 2020 | Low | Some concerns | Low | High | Some concerns | High |
Risk of bias for non-randomized trials according to ROBINS-I
Study | Risk of bias due to confounding factors | Risk of selection bias | Risk of intervention classification bias | Risk of bias due to deviation from protocolized interventions | Risk of bias due to missing data | Risk of bias due to outcome measurement | Risk of bias due to outcome selection | Total risk of bias |
---|---|---|---|---|---|---|---|---|
Mayberry et al. 2017 | Low | Low | Low | Low | Severe | Moderate | Low | Severe |
Raiff et al. 2016 | Moderate | Severe | Low | Low | Moderate | Moderate | Low | Severe |
The mHealth strategies implemented to improve adherence in DM II patients were similar to those described in other systematic reviews on the impact of Telemedicine on adherence to drug therapy. Interventions generally involved phone calls, SMS reminders, educational SMSs, and automated response systems, in line with the results of this review49,50. The use of mobile apps as a Telemedicine strategy in DM II patients was assessed in the systematic review by Cui et al., who only found a study assessing their impact on drug therapy24,48. The use of mobile apps in DM II patients is challenging, since this condition most frequently affects older adults, who are not as familiar to the use of mobile phones and technology as younger adults. This situation is changing, as the use of mobile phone is increasing among older adults. Therefore, further studies are necessary to assess improvements in adherence induced by the use of mobile apps adapted to the needs of this age group51,52.
One of the strategies found in this study was the use of incentives combined with SMS reminders. This strategy has been tested before in patients with infectious diseases and hypertension, and the results obtained in our study demonstrate that they could be effective in DM II patients53–55. Although the incentive plus SMS reminder strategy had a significant impact on adherence in the two studies, it is worth mentioning that the Raiff et al. study only included three patients, resulting in a high risk of bias. Further studies are warranted to draw conclusions on the effectiveness of this type of strategy in improving treatment adherence42.
The three studies involving a SMS-based strategies alone did not reveal any significant impact on adherence, unlike the strategies used by Bingham et al. or Fenerty et al. The latter report a positive effect of SMS-based strategies on adherence in most of the studies50,56. This inconsistency may be explained by the fact that baseline adherence exceeded 80% in the study by Gatwood et al., a rate that hardly can be improved44. The same applies to the Mayberry et al. study, where subjects reported full adherence in 6.1 ± 1.2 days of the last 7 days, which is in line with an adherence above 80%45. The results obtained by Sugita et al. may be due to the lack of a control group not involved in any mHealth-based strategy, since the control group also received SMS reminders43. These results indicate that intervention groups should only include patients with baseline treatment adherence problems, for researchers to be able to detect the positive effects of interventions. Otherwise, the number of subjects included should be increased significantly to be able to identify differences between the intervention and the control group.
Of the three studies which strategy included phone calls, only the one conducted by Odegard et al. had a significant impact on adherence47. These results are consistent with the ones obtained by Cassimatis et al., who reviewed eight studies involving educational phone calls to DM II patients. Statistically significant differences in treatment adherence were observed in three studies57. Bingham et al. included seven studies in patients with a diagnosis of DM, hypertension or dyslipidemia who received an intervention based on phone calls. In four studies, the intervention improved adherence significantly50. According to the authors, the interventions that are effective in improving adherence are focused on approaching patient-related barriers, which can be the key to their success. In our case, only the Odegard et al. strategy included person-to-person phone calls (non-automated), following the 4 A's model ('ask, advise, assist, arrange’)47.
It is worthy of note that this review did not include any strategy based on telementoring, where a patient with experience in the same intervention provides support and counseling to another. In the review conducted by Lee et al., several studies were found to assess the impact of different Tele-medicine-based strategies on glycemic control and cardiovascular risk16. Telementoring could be tested in DM II to improve drug adherence and put patients with adherence problems in contact with experienced patients through a health education program, as the one currently implemented in Catalonia58.
With respect to mobile apps-based strategies, Huang et al. report a significant impact on adherence, which is not consistent with the results of Bingham et al., where the two studies reviewed do not show any improvement in adherence in patients with chronic diseases48,50. These studies were not randomized, and the level of evidence they generated is below the one reported in this review. In addition, Bingham et al. report a baseline adherence > 85%, which makes it more difficult to detect variability in adherence. In the study performed by Patel et al. in patients with hypertension and high cardiovascular risk, subjects had a challenging socioeconomic status, and adherence worsened significantly after the intervention had been completed, which indicates apps-dependence50,59. There are several reviews and meta-analyses assessing the role of apps in drug adherence, with positive results in different patient populations with chronic diseases, which warrants further studies60–62.
The types of interventions found in this study are in line with the findings of other authors reporting that most Telemedicine interventions aimed at improving health outcomes and drug adherence are behavioral or educational16,23.
The effectiveness of the different types of interventions in improving adherence in patients with chronic diseases such as DM II has been assessed in a range of systematic reviews, with variability of results. In our case, a clear pattern by type of intervention was not observed, which is consistent with the review published by González-Bueno, who evaluated different types of interventions in plurimorbid patients. Thus, González-Bueno found no differences between educational, behavioral or other interventions63. The meta-analysis performed by Demonceau et al. revealed that interventions with an educational and/or feedback component are more effective than other interventions64. In some cases, the lack of consistency in the type of intervention and adherence assessment method hinder the performance of meta-analyses to determine whether some interventions are more effective than others65,66. Reviews consistently reveal that interventions tailored on the basis of patient needs and characteristics and mixed, hybrid or multimodal interventions are the most effective.
With respect to factors contributing to drug adherence, we found high variability in the socioeconomic status and educational level of patients. As poor health literacy and high treatment cost have been associated with low adherence, the studies assessing the impact of m-Health based interventions using this parameter should record and assess the level of health literacy and socioeconomic status of the subjects included in the study.
The sample of patients older than 60 years was small, probably due to the fact that they are less likely to use mobile phones, as indicated by Vervloet et al.41.
The follow-up period was generally short (< 6 months in 50% of studies), which is in line with previous studies on the effectiveness of Telemedicine in DM patients. Therefore, more studies are needed to assess the long-term impact of mHealth on adherence in patients with chronic conditions such as DM II16,24,57.
With regard to the limitations of this review, it is difficult to establish a relationship between the different mHealth strategies and their impact on adherence, due to the limited number of studies found, added to the small study samples. In addition, studies should exclude patients with disabilities, mental illness, cognitive impairment, or advanced age, which limits the generalization of results to the target population, considering that these factors are associated with drug adherence. Baseline adherence rates were already high in some studies, which makes it difficult to assess the impact of the interventions44,47. Finally, performing randomized, blinded, controlled clinical trials when the intervention involves a mHealth-based strategy is challenging, due to the risk of bias it generates.
The mHealth strategies found in the literature aimed at improving adherence to drug therapy in DM II patients included phone calls, SMS reminders, educational SMSs, electronic dispensing systems, automated response systems and mobile apps.
The impact of these strategies on drug adherence is variable, and conclusions cannot be drawn on the most effective intervention in DM II patients. However, electronic dispensing systems used in combination with SMS have been demonstrated to improve adherence.
Interventions were educational, behavioral or mixed, without any pattern having a greater impact on drug adherence.
The samples are not representative of some patient populations, since older adults are underrepresented and patients with cognitive impairment or sensory disabilities were excluded from most of the studies.
The risk of bias in studies on mHealth in DM II patients raises concerns in relation to the risk of bias due to deviation from the interventions, because of investigator's and/or patient's awareness of the study arm they have been allocated to.
Further studies are needed to identify the most effective mHealth strategies in improving treatment adherence in DM II patients.
FundingNo funding.
Conflict of interestsNo conflict of interests.
- 1.
'telemedicine’ AND 'diabetes mellitus’ AND 'medication compliance’
- 2.
'telemedicine’ AND 'non insulin dependent diabetes mellitus’ AND 'medication compliance’
- 3.
'mhealth’ AND 'diabetes mellitus’ AND 'medication compliance’
- 4.
'mhealth’ AND 'non insulin dependent diabetes mellitus’ AND 'medication compliance’
- 5.
'mobile health application’ AND 'diabetes mellitus’ AND 'medication compliance’
- 6.
'mobile health application’ AND 'non insulin dependent diabetes mellitus’ AND 'medication compliance’
- 1.
(mhealth OR telemedicine OR mobile health) AND (“diabetes mellitus” OR “diabetes mellitus, type 2”) AND (“medication adherence” OR “drug adherence”)
- 2.
((“Medication Adherence”[Mesh] AND ((y_10[Filter]) AND (clinicaltrial[Filter] OR randomizedcontrolledtrial[Filter]))) AND ((“Diabetes Mellitus, Type 2”[Mesh]) OR “Diabetes Mellitus”[Mesh])) AND (“Telemedicine”[Mesh] OR “Mobile Health” OR “Health, Mobile” OR “mHealth” OR “Telehealth” OR “eHealth” AND ((y_10[Filter]) AND (clinicaltrial[Filter] OR randomizedcontrolledtrial[Filter])))
- 3.
((“Medication Adherence”[Majr] AND ((y_10[Filter]) AND (clinicaltrial[Filter] OR randomizedcontrolledtrial[Filter]))) AND ((“Diabetes Mellitus, Type 2”[Majr]) OR “Diabetes Mellitus”[Majr])) AND (“Telemedicine”[Majr] OR “Mobile Health” OR “Health, Mobile” OR “mHealth” OR “Telehealth” OR “eHealth” AND ((y_10[Filter]) AND (clinicaltrial[Filter] OR randomizedcontrolledtrial[Filter])))
- 4.
((“Text Messaging”[Mesh]) AND “Diabetes Mellitus”[Mesh]) AND “Medication Adherence”[Mesh]
- 5.
((“Wearable Electronic Devices”[Mesh]) AND “Diabetes Mellitus”[Mesh]) AND “Medication Adherence”[Mesh]
- 1.
((telemedicine OR mHealth) AND (diabetes mellitus OR type 2 diabetes OR non insulin dependent diabetes mellitus) AND (medication adherence OR medication compliance))
- 1.
allintitle: “telemedicine” OR “mhealth” OR “mobile technologies” OR “telehealth” OR “mobile health”+”diabetes” OR “diabetes mellitus” OR “type 2 diabetes” +”medication compliance” OR “medication adherence” OR “Treatment Adherence and Compliance” OR “adherence” OR “drug adherence”