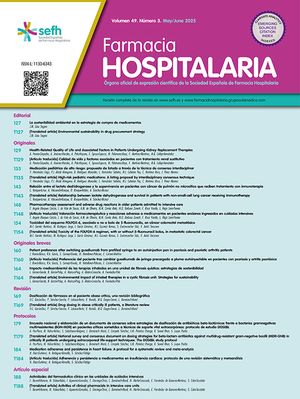
The consolidation of Telepharmacy during the COVID-19 pandemic has raised the need for managing large volumes of real-time activity data through data analysis. The aim of this project was to design a dynamic, user-friendly, customizable scorecard in a hospital pharmacy service for the visualization and analysis of Telepharmacy activity indicators through the use of advanced business intelligence technology.
MethodThe software tool was developed by a multidisciplinary team between April and May 2021, driven from the hospital pharmacy service. Once the Telepharmacy indicators of interest were established, datasets were extracted from raw databases (administrative databases, Telepharmacy database, outpatient dispensing software, drug catalogues) through data analysis. The different data sources were integrated in a scorecard using PowerBI®. The criteria for processing missing and duplicated data were defined, and data pre-processing, normalization and transformation were performed. Once the pilot scorecard was validated by different profiles of users, the structure was designed for the panels to automatically update as databases were updated.
ResultsDesign and implementation of a scorecard of Telepharmacy activity: general descriptive panel (demographic profile of patients, count and delivery conditions, program and medical service); geolocation of destination; pharmacological profile; relative analysis of patients involved in the Telepharmacy program with respect to the total of outpatients. In the last updating as of January 2022, data from 16,000 dispensations to more than 4,000 patients had been collected. This means that 21.93% of outpatients had benefited at some time point from the Telepharmacy service. Filters enable the visualization of timeline progress and patient characterization, and measure Telepharmacy activity by program.
ConclusionsThe processing of large Telemedicine datasets from various sources through Business Intelligence in a hospital pharmacy service makes it possible to synthesize information, generate customized reports, and visualize information in a dynamic and attractive format. The application of this new technology will help us improve strategic clinical and management decision making.
La consolidación de la Telefarmacia en el contexto de la pandemia por la COVID-19 exige manejar a tiempo real un gran volumen de datos de actividad mediante análisis de datos. El objetivo de este trabajo fue diseñar un cuadro de mando ágil, personalizable y dinámico para la visualización y análisis de indicadores de actividad en Telefarmacia en un servicio de farmacia de hospital, mediante el empleo de herramientas avanzadas de inteligencia empresarial (business intelligence).
MétodoUn equipo de trabajo multidisciplinar desarrolló una herramienta de software entre abril y mayo de 2021 impulsado desde el servicio de farmacia de hospital. Una vez consensuados los indicadores de interés en Telefarmacia, se extrajeron los datos a partir de bases de datos brutas (base de datos de Telefarmacia, programa de dispensación de pacientes externos, bases de datos administrativas, catálogos de fármacos) mediante análisis de datos. La integración de las diferentes fuentes de datos en el cuadro de mando se realizó mediante PowerBI®. Se definió el manejo de los datos perdidos y duplicados y se aplicó preprocesamiento, normalización y transformación de los datos. Una vez validado el piloto por diferentes tipos de usuarios, se diseñó la estructura para actualización automática de los paneles con las sucesivas actualizaciones de las fuentes de datos.
ResultadosDiseño e implementación de un cuadro de mando de la actividad en Telefarmacia: panel descriptivo general (perfil demográfico de pacientes, recuento y condiciones de envíos, programa y servicio médico); geolocalización de destino; perfil farmacológico; análisis relativo de los pacientes beneficiarios de Telefarmacia respecto del total de pacientes externos. En el último corte, a enero de 2022, se habían incluido datos de 16.000 dispensaciones con entrega informada a más de 4.000 pacientes, lo que supone que el 21,93% de los pacientes externos han estado en algún momento en el programa de Telefarmacia. La aplicación de filtros permite visualizar la evolución temporal, caracterizar grupos de pacientes y dimensionar la actividad por programas.
ConclusionesEl procesamiento de paquetes de datos de Telemedicina, de gran volumen, difícil manejo y procedentes de diversas fuentes relativas a Telefarmacia mediante inteligencia empresarial, en un servicio de farmacia de hospital, permite sintetizar la información y proporcionar informes personalizados y visualizaciones dinámicas y atractivas. La aplicación de estas nuevas tecnologías puede ayudarnos a mejorar la toma de decisiones estratégicas, tanto clínicas como de gestión.
The SARS-CoV-2 pandemic expedited the development of Telepharmacy, which was emerging as a new pharmaceutical care model in the national1,2 and international3–6 level. Telepharmacy evolved as new restrictions were imposed in hospitals to ensure the continuity of high-quality, cost-effective pharmaceutical care. As a result, the Spanish Society of Hospital Pharmacy (SEFH) has incorporated the implementation of Telepharmacy in hospital pharmacies in Spain7 as a new strategic line8–10.
In the case of the Hospital Pharmacy Service of Hospital Clínico San Carlos (HCSC), a technology-based Telepharmacy Service was set up in a few days. Data of candidate patients for inclusion in the Telepharmacy program was entered onto a groupware spreadsheet that allowed real-time edition by different users. Then, information was entered onto a password-protected Excel book to support teleconsultations and the functionalities required, i.e. automatic generation of informed consents, work-up lists, shipping and rescheduling records, as appropriate. Dispensations were recorded using the Farmatools® system.
Initially, the Telepharmacy record system had some limitations. At technical level, data mining was not feasible with the equipment and resources available. Data bases were compartmentalized into different formats. Their structure and contents were not always correctly categorized due to human or technical errors or to the characteristics of the system itself. In addition, data processing had to comply with personal data protection laws.
In a context of volatility, uncertainty, complexity, and ambiguity (VUCA) exacerbated by the COVID-19 pandemic, technological tools are required to support real-time strategic decision-making in healthcare organizations. These technologies enable the detection of system connections, behaviors, and weaknesses to reduce uncertainty. In the era of Big Data, the use of intelligent data mining results in improved decision making and enhanced clinical outcomes11. The generation of massive data requires system interoperability and synthetization of relevant information. At management level, resource optimization strategies are developed based on advanced data analytics, patient prioritization or stratification criteria, early detection and correction of imputation errors, and resource-use planning, among other strategies. In addition, fluent coordination and communication among healthcare professionals facilitates the evaluation of clinical outcomes from a multidisciplinary approach. The availability of healthcare quality assessment tools based on process/result indicators is crucial to enhancing integrated patient care.
Information about Hospital Pharmacy patients is distributed across multiple information systems. The COVID-19 pandemic raised the need for an institutional data repository that contained information about healthcare activity, clinical variables, and patient monitoring. To meet this need, Hospital Clínico San Carlos set up a clinical data bank (BDCLIN_HCSC) promoted by the Innovation Unit of the Health Research Institute of Hospital Clínico San Carlos (IdISSC). The BDCLIN_HCSC is an information interaction model that enables data mining for clinical, administrative, and research purposes that also facilitates the development of decision-making support models. The Hospital Pharmacy Service database is included in BDCLIN_HCSC as a shared service for all clinical areas of the hospital. The Institutional Review Board approved the BDCLIN_HCSC project and associated subprojects.
The use of scorecards of Telepharmacy indicators helps assess relevant aspects such as healthcare activity, clinical effectiveness, logistics, patient or professional experience, and economic evaluation of results12. Scorecards built on business intelligence provide population data, allow the health parameters monitoring, and support clinical and strategic decision making13. Massive datasets are displayed in attractive tables, graphs, diagrams and quantitative information. The reports generated are dynamic, and parameterizable according to end-user's needs.
The operating procedures established by the Innovation Unit in relation to the BDCLIN_HCSC system allowed to solve some of the problems detected in the initial Telepharmacy record system. Thus, a parameterizable scorecard was designed to enable the accomplishment of existing and future needs. This project was aimed at designing an easy-to-use, customizable, dynamic scorecard for the visualization and analysis of Telepharmacy activity indicators. This scorecard was focused on dispensing and informed delivery of medications through the use of business intelligence technologies.
MethodsA multidisciplinary Working Group was created including professionals from the HCSC Pharmacy Service and the IdISSC Innovation Unit i.e. biomedical engineers, managers, practitioners and hospital pharmacists. Firstly, the Telepharmacy activity indicators to be parameterized were established based on a literature review, face-to-face/online meetings, and quality department counseling. Indicators were regularly re-evaluated after an analysis of results.
Once Telepharmacy care indicators were defined, datasets were extracted from administrative databases, dispensing and informed delivery (DID) records, and the pharmacy information system (Farmatools®). Only relevant tables or associations were selected for visualization (in Excel format, *.xlsx). Firstly, data on the sex and date of birth of patients was collected from administrative databases. In March 2020, the Hospital Pharmacy Service built and managed the DID database. Then, the DID database was used to design the Telepharmacy table (TP-HPS), which provided data such as date of dispensing and delivery, patient data, and name and relevant characteristics of the drug to be dispensed, to ensure medications were delivered in optimal safety conditions. Finally, from the third source of data, Farmatools®, another two tables were generated, as follows: the Outpatient Dispensing Table, and the Pharmaceutical Specialty-Active Ingredient-ATC Code Correspondence Table. The Outpatient Dispensing Table shows all dispensations (in-person or remotely) performed by the Hospital Pharmacy Service for a specific time period (date, patient, dispensing service and program). The Pharmaceutical Specialty-Active Ingredient-ATC Code Correspondence Table shows relevant information on the medications available for prescription. Services and programs are categorized according to criteria established by managers and practitioners to facilitate interactive visualization by ATC group and active ingredient. Figure 1 shows the relational diagram of information sources used for the scorecard version of January 2022.
Through teamwork, the design of the tool was adapted to meet the needs detected by the Hospital Pharmacy Service. In addition, the multidisciplinary approach adopted allowed to analyze the distribution and structure of the databases available within their clinical context. Data cleaning techniques were used to separately adapt information sources to the needs identified. Once databases were free of noise, inconsistencies, abnormalities and duplicates, data integration techniques were applied to merge them into a single database. Data transformation techniques were also implemented to calculate age, group services and programs, and perform the relative estimation of in-person dispensations vs Telepharmacy dispensations. Then, additional data transformation and reduction techniques were applied to the overall data set to enable data mining through business intelligence technology.
The Power BI® tool was used for the visualization of data and the development of an interactive scorecard. The first version of the scorecard was developed between April and May 2021 for the March 2020-March 2021 period. The first prototype was designed based on indicators that were defined by integrating all sources of information. Architecture design allows to sequentially incorporate data sets including clinical variables, laboratory parameters or cost variables, among others, resulting in the generation of a set of interconnected tables.
The multidisciplinary team used the iterative methodology (design, development, validation) to adjust the design and content of the scorecard by adding new panels and indicators.
The scorecard was designed based on the data minimization principle, by which only essential variables are selected for implementation. Aggregated datasets are visualized by program, service, or ATC group. Individual patient variables were excluded to prevent patient identification.
During the third phase of the iterative methodology, the tool was validated at functional and clinical level by four user categories: biomedical engineers, administrative personnel, hospital pharmacists, and managers. Validation was based on accomplishment of the needs identified, in terms of interoperability with other information sources, simplicity, and user experience.
Once the tool was validated, it was designed to automatically update as data sources were updated and prepared for scalability, otherwise said, information was structured for easy adaptation to data accrual without it involving any loss of accuracy or quality. Thus, databases were designed to have a static structure, considering the type, name and distribution of variables in information sources. Then, they were fragmented by month, so that they could be integrated with the data generated in the following months, to optimize time and storage capacity. This way, panel size is regularly updated without it requiring to modify visual object design and distribution.
ResultsWe used three sources of data, 19 variables from a total of 90, and 8 recodifications/stratifications for categorical variables. A total of 2,527,406 data rows were processed. Following iterative methodology, three validations of the scorecard were performed, as new needs were identified or improvements in the use of the technology were adopted.
The final scorecard model consists of five interrelated panels:
- •
General descriptive panel: demographic profile of Telepharmacy users, shipping volume and conditions, program, and HCSC medical service (Figure 2).
- •
Panel of dispensing and informed delivery by geographic distribution, including destination geolocation.
- •
Two relative panels of prescription profile by medical service, HPS program and relative frequency, stratified by age range, date, pharmaceutical presentation, pharmaceutical form, and ATC group.
- •
Comparative panel of Telepharmacy activity and total of dispensations delivered to HPS outpatients (Figure 4).
Between 25/03/2020 and 31/01/2022 a total of 16,007 dispensations and informed deliveries (DIDs) were provided to 4,050 different patients. As many as 33.57 % of deliveries included refrigerated medications (cold chain). Mean age of patients (49.75 % female) was 62.35 ± 16.44 years (Figure 2).
During this period, the patients included at least once in the Telepharmacy program accounted for 21.93 % of the total of HPS outpatients. Figure 3 shows a comparative description of the dispensations performed by Telepharmacy as compared to face-to-face dispensations. In April 2020, the volume of home DIDs (3,054) exceeded face-to-face deliveries (2,239) and remained stable from the Fall of 2020.
Overall, the ratio of DIDs per patient was 3.95. The most frequent ATC groups were group L (40.35%) and group J (26.67 %). Telepharmacy users were primarily patients of the service of Neurology (1,028 patients), Internal Medicine (827) and Reumatology (446).
The prescription panel shows timeline progress (Figure 4). During the first trimester, 40.5% of patients had been included in the Telepharmacy program at least once, whereas in the last trimester, this percentage reached 4.7% The profile of medications dispensed changed over time. Although the volume of dispensations for multiple sclerosis and, to a lesser extent, HIV, remained stable, the percentage of active ingredients for master formulas increased.
DiscussionBusiness intelligence technology helps users visualize and understand mass amounts of complex data13. Our scorecard provides effective access to large volumes of data related to Telepharmacy activity, enables consistent analysis, and reduces variability, which generates a relevant added value, as it can be applied to routine practice. The scorecard also simplifies early detection of imputation or source data parameterization errors, and facilitates the identification of potential improvement areas, with several applications in quality control.
Tableau® and Microsoft Power BI® are the most widely used data visualization tools in healthcare, although the use of Tableau® in the healthcare industry and hospitals is still limited14. Microsoft Power BI® is a versatile tool compatible with different types of databases and graphic applications that simplifies data analysis and enables real-time database updating15. It is available free of charge at the portfolio of applications of our Regional Department of Health and can be accessed from all hospital computers and user profiles.
Its intuitive interface provides an optimal end-user experience and does not require advanced training in the use of the tool, as it occurs with tools such as Microsoft Excel/Access®. The availability of customized filters allows users to edit panel information according to their interests and generate customized reports for each consultations in just a few seconds. For example, this tool helps identify monitoring trends over time, parameterize patient selection criteria (simultaneous inclusion in two programs, addition of filters) or detect patient profile patterns for patient characterization, which can be applied to patient stratification.
The design of the scorecard facilitates critical analysis and research16. For example, the Telepharmacy prescription panel stratified by medical service, therapeutic program and active ingredients enables the analysis of percentage increases in the number of treatments for chronic pain or pediatric rare conditions, which reflects Pharmacy Hospital Service's commitment to the humanization of frail patient care.
The scorecard design is based on hospital needs and characteristics (12-h face-to-face consultation hours in working days, geographic concentration, healthcare model with limited implementation of Telemedicine, patient home as the primary point of delivery, etc.). The prioritization criteria established by patient profile have moved to balance synchronic face-to-face and telematic care involving DID. In any case, structure is designed to adapt to other indicators and enable benchmarking between clinical services and institutions. Publication on a web URL provides simultaneous access to several users, which promotes result transparency and dissemination to improve quality.
The SEFH published the “Scorecard of Telepharmacy Indicators” handbook to establish a conceptual framework for measuring Telepharmacy indicators12. Our scorecard allows the estimation of healthcare and logistics indicators. For example, indicators show how the healthcare model has changed its focus to specific patient groups to ensure the sustainability of the system:
- •
Indicator 31. Patients included in the Telepharmacy DID program for Hospital Pharmacy Service outpatients: 28.58% in 2020 vs 7.41% in 2021.
- •
Indicator 40. DIDs performed per patient: 3.03 in 2020 vs 3.98 in 2021.
Our group is working on the parameterization of additional indicators as suggested in the handbook, including indicators that discriminate by scope of application, scheduled consultations vs emergency consultations, cost study, and event record. Indicators evolve as our Telepharmacy activity does. Hence, new procedures and variables of interest have been incorporated to assess patient adherence and experience17. As Telepharmacy is continuously evolving, the publication of experiences and tools adapted to the context of each hospital pharmacy service contributes to generating evidence and defining the future of Telepharmacy.
A limitation of this model is that the scorecard feeds from data discharges and updating depends on the regularity of discharges. However, it is designed to automatically update and requires minimal manual processing. The quality of data sources is also a methodological limitation. The pre-processing techniques applied minimize potential between-subject variability or human errors in data recording. The difficulty in categorizing variables with non-standardized names was overcome by detecting mismatched categories and relating them to each other by mapping.
The next phases of the project and future research will be aimed at developing a database that allows structured collection of Telepharmacy data based on quality and traceability criteria. The database will enable the collection of patient reported outcomes (PROs) with all data protection and security guarantees in a format that is compatible with the existing scorecard.
Additionally, new databases are being incorporated, including laboratory data (viral load) and clinical data (ED admissions, hospitalization, minimum data set, primary and secondary diagnosis at discharge) to complement management reports with clinical outcomes.
Further development may help establish patient journey and improve healthcare pathways based on suitability and effectiveness criteria. The most advanced level contains modeling and simulation techniques.
Business Intelligence technology enables the processing of massive data sets from different sources. Based on artificial intelligence, the scorecard synthesizes data, provides dynamic, attractive visualizations and generates interactive reports for a more effective communication of results and data analysis. These technologies will improve strategic clinical and management decision making by enabling users to analyze healthcare activity, characterize/select patients, identify interactions, trends, and points of weakness of the system, allocate resources, and perform long-term follow-up.
FundingThis project was partially funded by UCB S.A. in collaboration with Instituto de Investigación Sanitaria San Carlos, and the Intensification Program of the Fundación para la Investigación Biomédica del Hospital Clínico San Carlos, in collaboration with the Regional Health System of Madrid.
AcknowledgementsWe thank Antonio Daniel Prieto Prieto for his review and disinterested contributions.
Conflict of interestsNo conflicts of interest.
Presentation at congressesThe first version of the dashboard described in this paper (May 2021) was presented at the 66th Congress of the SEFH in the category of “Perla farmacéutica” under the title “To BI or not to be”, which was selected for presentation as an oral communication in virtual format on October 21, 2021. All data are original and authentic.
Contribution to scientific literature
A description is provided of the design and implementation of a dynamic tool based on business intelligence for real-time analysis of data on Telepharmacy activity in a Hospital Pharmacy Service.
Customized scorecard panels enable the processing of data from interconnected databases to support clinical decision making in routine practice.